Get new posts by email or rss feed
About three months ago, Scott Alexander from Astral Codex Ten, posted an observational study looking at his performance on WordTwist as a function of CO2 level. In a dataset of ~800 games, he saw no correlation between his relative performance (vs. all players) and CO2 levels (R = 0.001, p = 0.97).
This was in stark contrast to a study by Fisk and co-workers, that found that CO2 levels of 1,000 and 2,500 ppm significantly reduced cognitive performance across a broad range of tasks.
I was really interested to see this. Back in 2014, I started a company, Mosaic Materials, to commercialize a CO2 capture material. At the time, a lot of people I talked with were excited about this study, but I was always really suspicious of the effect size. Since then, studies have come out that both did and did not observe this effect, though the lack of greater follow up further increased my skepticism.
In addition to being curious regarding the effect of CO2 on cognition, I found the idea of using simple, fun games to study cognitive effects to be extremely interesting. Since even small cognitive effects would be extremely important/valuable, a quick, fun to use test like WordTwist would allow for the required large dataset.
I don’t enjoy word games, but Scott pointed to a post on LessWrong by KPier that suggested using Chess, which I play regularly. Actual games seemed too high variance and time consuming, but puzzles seemed like a good choice.
Based on all that, I got a CO2 meter and started doing 10 chess puzzles every morning when I woke up, recording the CO2 level in addition to all my standard metrics. So far, I have ~100 data points, so I did an interim analysis to see if I could detect any significant correlations.
Here’s a summary of what I found:
- Chess puzzles are a low effort (for me), but high variance and streaky measure of cognitive performance
- Note: I didn’t test whether performance on chess puzzles generalizes to other cognitive tasks
- No statistically significant effects were observed, but I saw modest effect sizes and p-values for:
- CO2 Levels >600 ppm:
- R2 = 0.14
- p = 0.067
- Coefficient of Variation in blood glucose
- R2 = 0.079
- p = 0.16
- CO2 Levels >600 ppm:
- The current sample size is underpowered to detect the effects I’m looking for. I likely need 3-4x as much data to reliably detect the effect sizes I’m looking for.
- Given how many correlations I looked at, the lack of pre-registration of analyses, and the small number of data points, these effects are likely due to chance/noise in the data, but they’re suggestive enough for me to continue the study.
Next Steps
- Continue the study with the same protocol. Analyze the data again in another 3 months.
Questions/Requests for assistance:
- My variation in rating has long stretches of better or worse than average performance that seem unlikely to be due to chance. Does anyone know of a way to test if this is the case?
- Any statisticians interested in taking a deeper/more rigorous look at my data or have advice on how I should do so?
- Any suggestions on other quick cognitive assessments that would be less noisy?
– QD
Details
Purpose
- To determine if any of the metrics I track correlates with chess puzzle performance.
- To assess the usefulness of Chess puzzles as a cognitive assessment.
Background
About three months ago, Scott Alexander from Astral Codex Ten, posted an observational study looking at his performance on WordTwist as a function of CO2 level. In a dataset of ~800 games, he saw no correlation between his relative performance (vs. all players) and CO2 levels (R = 0.001, p = 0.97).
This was in stark contrast to a study by Fisk and co-workers, that found that CO2 levels of 1,000 and 2,500 ppm significantly reduced cognitive performance across a broad range of tasks.
I was really interested to see this. Back in 2014, I started a company, Mosaic Materials, to commercialize a CO2 capture material. At the time, a lot of people I talked with were excited about this study, but I was always really suspicious of the effect size. Since then, studies have come out that both did and did not observe this effect, though the lack of greater follow up further increased my skepticism.
In addition to being curious regarding the effect of CO2 on cognition, I found the idea of using simple, fun games to study cognitive effects to be extremely interesting. Since even small cognitive effects would be extremely important/valuable, a quick, fun to use test like WordTwist would allow for the required large dataset.
I don’t enjoy word games, but Scott pointed to a post on LessWrong by KPier that suggested using Chess, which I play regularly. Actual games seemed too high variance and time consuming, but puzzles seemed like a good choice.
Based on all that, I got a CO2 meter and started doing 10 chess puzzles every morning when I woke up, recording the CO2 level in addition to all my standard metrics. So far, I have ~100 data points, so I did an interim analysis to see if I could detect any significant correlations.
Results & Discussion
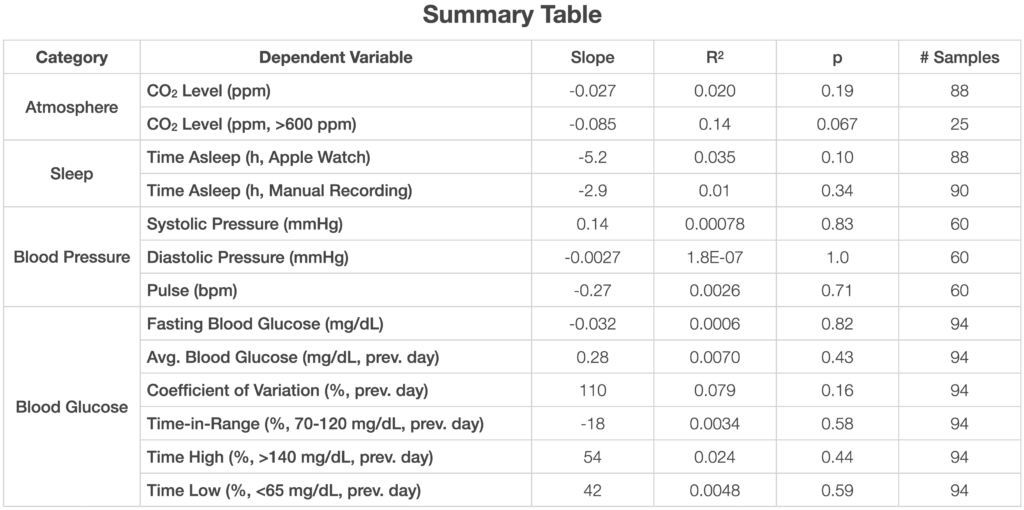
Performance vs. Time
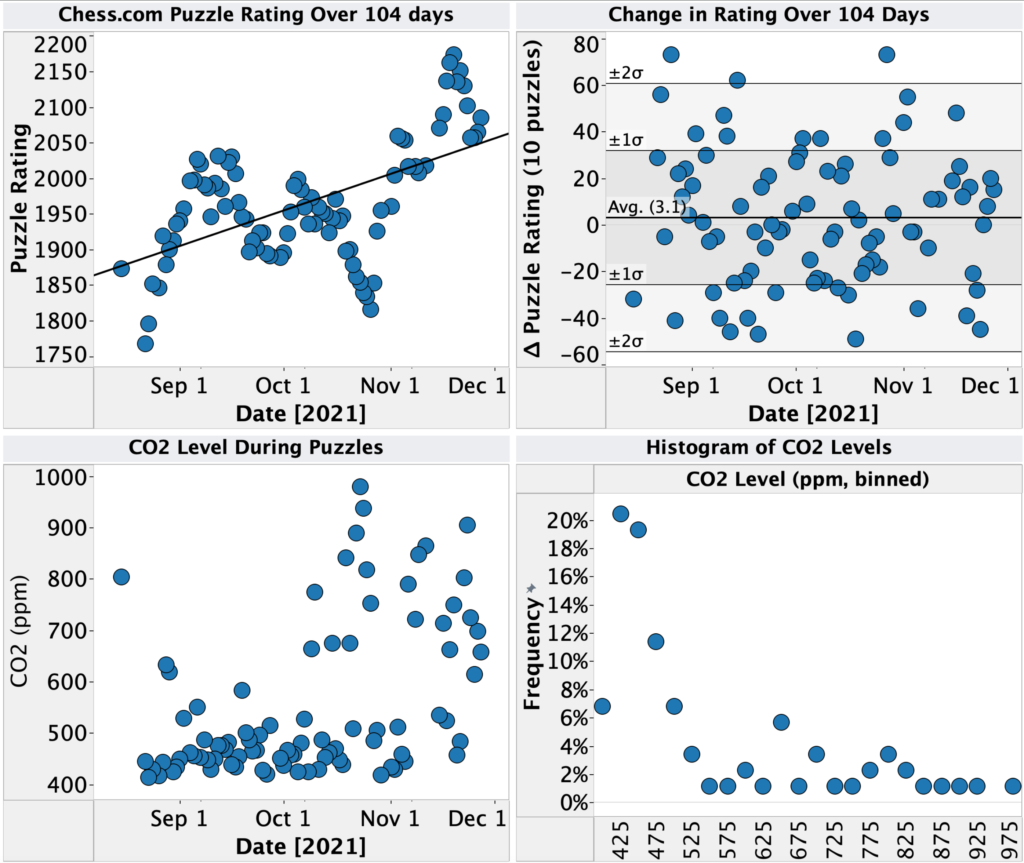
Before checking for correlations, I first looked at my puzzle performance over time. As shown above (top left), over the course of this study, my rating improved from 1873 to 2085, a substantial practice effect. To correct for this, all further analyses were done using the daily change in rating.
Looking at the daily change, we see a huge variation:
- Average = 3
- 1σ = 29
Moreover, the variation is clearly not random, with long stretches of better or worse than average performance that seem unlikely to occur be chance (does anyone know how to test for this?).
All this points to Chess puzzles not being a great metric for cognitive performance (high variance, streaky), but I enjoy it and therefore am willing to do it long-term, which is a big plus.
CO2 Levels
During the course of this study, CO2 levels varied from 414 to 979 ppm. Anecdotally, this seemed to be driven largely by how many windows were open in my house, which was affected by the outside temperature. Before October, when was relatively warm and we kept the windows open, CO2 levels were almost exclusively <550 ppm. After that, it got colder and we tended to keep the windows closed, leading to much higher and more varied CO2 levels.
Unfortunately for the study, the CO2 levels I measured were much lower than those seen by Scott Alexander and tested by the Fisk and co-workers. In particular, Fisk and co-workers only compared levels of 600 ppm to 1000 & 2500 ppm.
Given this difference in the data, I performed a regression analysis on both my full dataset and the subset of data with CO2 > 600 ppm. The results are shown below:
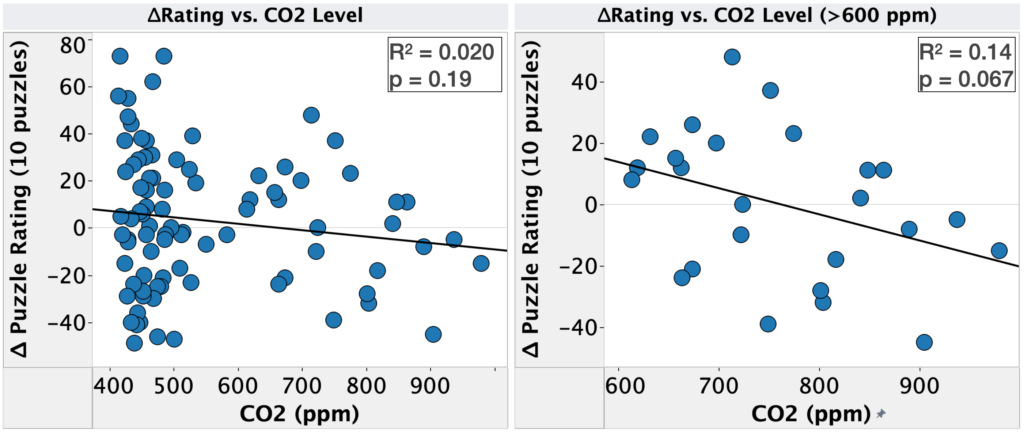
For the full dataset, I see a small effect size (R2 = 0.02) with p=0.19. Restricting to only CO2 > 600 ppm, the effect size is much larger (R2 = 0.14), with p = 0.067. Given how many comparisons I’m making, the lack of pre-registration of the CO2 > 600 ppm filter, and the small number of data points (only 27 samples with CO2 > 600), this is likely due to chance/noise in the data, but it’s suggestive enough for me to continue the experiment. We’ve got a few more months of cold weather, so I should be able to collect a decent number of samples with higher CO2 values.
Sleep
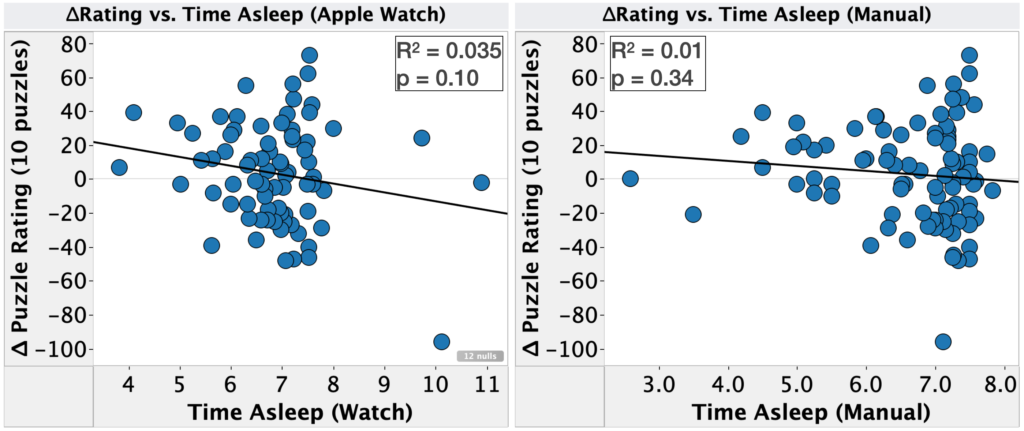
Since I had all this puzzle data, I decided to check for correlations with all the other metrics I track. Intuitively, sleep seemed like it would have a large effect of cognitive ability, but the data shows otherwise. Looking at time asleep from both my Apple Watch and manual recording, I see low R2 (0.035 & 0.01) with p-values of 0.10 and 0.34, respectively. Moreover, the trend is in the opposite direction as expected, with performance getting worse with increasing sleep.
I was surprised not to see an effect here. It’s possible this is due to the lack of reliability in my measurement of sleep. Neither the Apple Watch or manual recording are particularly accurate, which may obscure smaller effects. I have ordered an Oura Ring 3, which is supposed to be much more accurate. I’ll see if I can measure an effect with that.
The other possibility is that since I’m doing the puzzles first thing in the morning, when I’m most rested, sleep doesn’t have as strong an effect. I could test this by also doing puzzles in the evening, but not sure whether I’m up for that…
Blood Pressure & Pulse
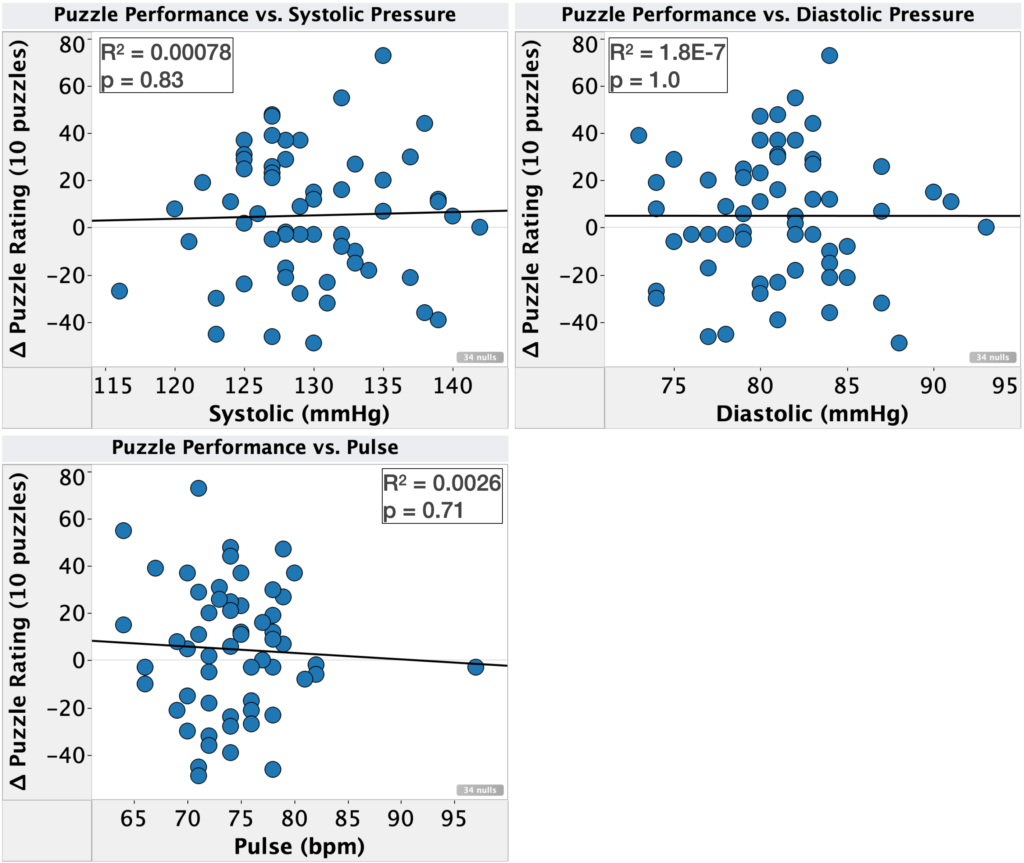
Not much to say for blood pressure. R2 was extremely small and p-values were extremely high for all metrics. Clearly no effect of a meaningful magnitude.
Blood Glucose
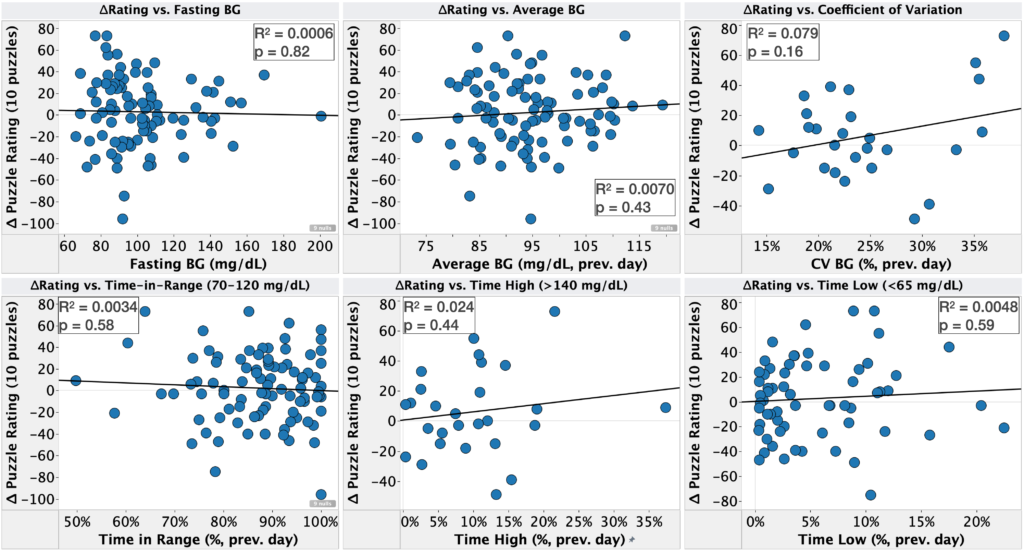
With the exception of coefficient of variation, no sign of an impact of blood glucose on puzzle performance (low R2, high p-value). For coefficient of variation, there was only a modest R2 of 0.079 and a p-value of 0.16. Still likely to be chance, especially with the number of comparisons I’m doing, but worth keeping an eye on as I collect more data.
Similar to sleep, I was surprised not to see an effect here. Low blood glucose is widely reported to impair cognitive performance, every doctor I’ve been to since getting diabetes has commented on low blood sugar impairing cognitive performance, and subjectively I feel as though I’m thinking less clearly when my blood sugar is outside my normal range and am worn out by it for a while after the fact.
All that said, as mentioned in the section on sleep, doing the puzzles first thing in the morning, when I’m most rested, might be masking the effect. The only way I can think to test this is to do puzzles in the evening, but that’s much less convenient.
Power Analysis
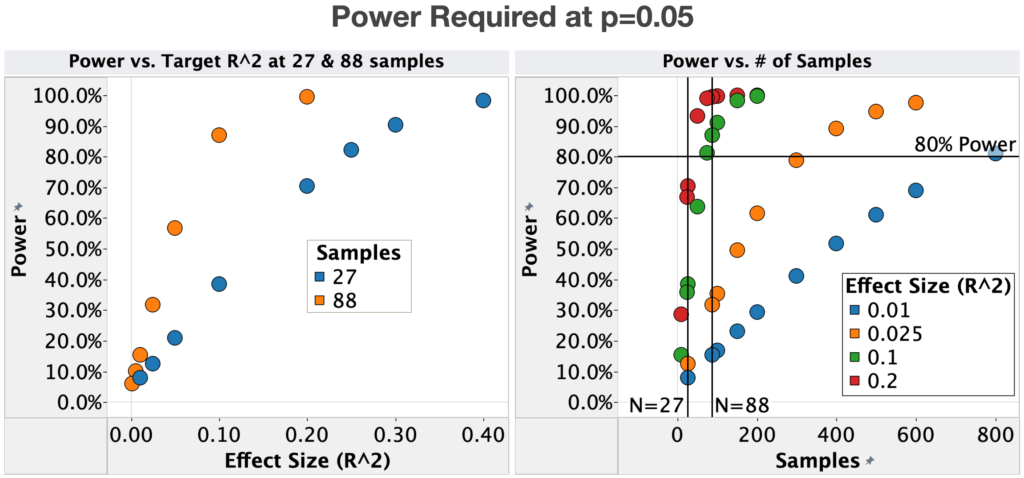
One concern with all of these analysis is whether the study had sufficient power to detect an effect. To check this, I looked at the statistical power at the sample and effect sizes that were seen.
For sample size, there were 100 total samples, 88 with CO2 measurements and 27 with CO2 levels >600 ppm. With 88 samples, there was a ~90% chance of detecting an R2 of 0.1, but this dropped to only ~40% with 27 samples. Given that R2 = 0.1 would be a practically meaningful effect size for the impact of natural variation in room atmosphere on cognitive ability, this indicates that it’s not surprising that the CO2 analyses did not reach statistical significance and that substantially more data is needed to rule out an effect.
In terms of detectable effect sizes, 88 samples gives a pretty good chance of detecting R2 = 0.1 (~90%), but the power drops rapidly below that, with an R2 of 0.025 having a power of only ~35%. Again, given the practical importance of cognitive performance, I’m interested in detecting small effect sizes, so it seems worthwhile to collect more data, especially as I enjoy the chess puzzles and am already collecting all the other metrics.
Conclusions & Next Experiments
Conclusions
- Chess puzzles are a low effort (for me), but high variance and streaky measure of cognitive performance
- Note: I didn’t test whether performance on chess puzzles generalizes to other cognitive tasks
- No statistically significant effects were observed, but I saw modest effect sizes and p-values for:
- CO2 Levels >600 ppm:
- R2 = 0.14
- p = 0.067
- Coefficient of Variation in blood glucose
- R2 = 0.079
- p = 0.16
- CO2 Levels >600 ppm:
- The current sample size is underpowered to detect the effects I’m looking for. I likely need 3-4x as much data to reliably detect the effect sizes I’m looking for.
- Given how many correlations I looked at, the lack of pre-registration of analyses, and the small number of data points, these effects are likely due to chance/noise in the data, but they’re suggestive enough for me to continue the study.
Next Steps
- Continue the study with the same protocol. Analyze the data again in another 3 months.
Questions/Requests for assistance:
- My variation in rating has long stretches of better or worse than average performance that seem unlikely to be due to chance. Does anyone know of a way to test if this is the case?
- Any statisticians interested in taking a deeper/more rigorous look at my data or have advice on how I should do so?
- Any suggestions on other quick cognitive assessments that would be less noisy?
– QD
Methods
Pre-registration
My intention to study the effect of CO2 on cognition was pre-registered in the ACX comment section, but I never ended up pre-registering the exact protocol or analysis.
Differences from the original pre-registration:
- I only used chess puzzles to assess cognition and did not include working memory or math tests.
- Evaluated other mediators (blood pressure, blood glucose, and sleep) in addition to CO2 levels.
Procedure
- Chess puzzles:
- Each morning, ~15 min. after I woke up, I played 10 puzzles on Chess.com and recorded my final rating.
- No puzzles were played on Chess.com at any other time, though I occasionally played puzzles on other sites.
- Manual measurements:
- Manual recording of sleep, blood pressure, and pulse was performed upon waking, before playing the chess puzzles.
- CO2 was recorded immediately after completion of the chess puzzles.
Measurements
- Chess puzzles: Chess.com iPhone app
- Sleep: Apple watch + Autosleep app
- Blood glucose: Dexcom G6 CGM
- Blood pressure & pulse: Omron Evolve
Analysis & Visualization
- Sleep and blood glucose data was processed using custom python scripts (sleep, Dexcom)
- Linear regression was performed using the analysis function in Tableau.
- Data was visualized using Tableau.
- Power calculations for linear regression were performed using Statistics Kingdom with alpha=0.05 and digits=10.